What is DeepHPI?
Host-pathogen protein-protein interactions (HPIs) plays vital roles among several biological processes. Furthermore, there is an interest in those interactions that are related with infectious diseases, which seems crucial to understand the mechanism behind the infection process per se and therefore, unravel potential targets to develop therapeutic approaches. Recently, efforts have been made to collect most of the data present in the literature about HPIs and algorithms to transfer that knowledge into unknown systems has been implemented. Beyond single-species protein-protein interaction (PPI) prediction, there is not a comprehensive analysis modelling those wide-range databases using machine learning methods, which have been proved efficient to summarize complex systems.
A comparison between different machine learning methods such as support-vector machines (SVM), artificial neural networks (ANN) and Deep Learning (CNN) was performed. Moreover, several sequence-based features were tested, including Autocorrelation, Dipep composition, Conjoint Triad, Quasi-order and One-hot. Models to predict HPI were generated by the combination of features and machine learning methods. The best models from this benchmark were implement in the DeepHPI webserver
Modelling protein-protein interactions in two-sided systems add an extra layer of complexity to the protein-protein prediction problem, Among the methods tested, Convolutional neural network looks promising and further architectures will need to be explored. We hope that from our comprehensive comparison the fundamentals in the prediction of host-pathogen interaction using machine learning techniques will be settled.
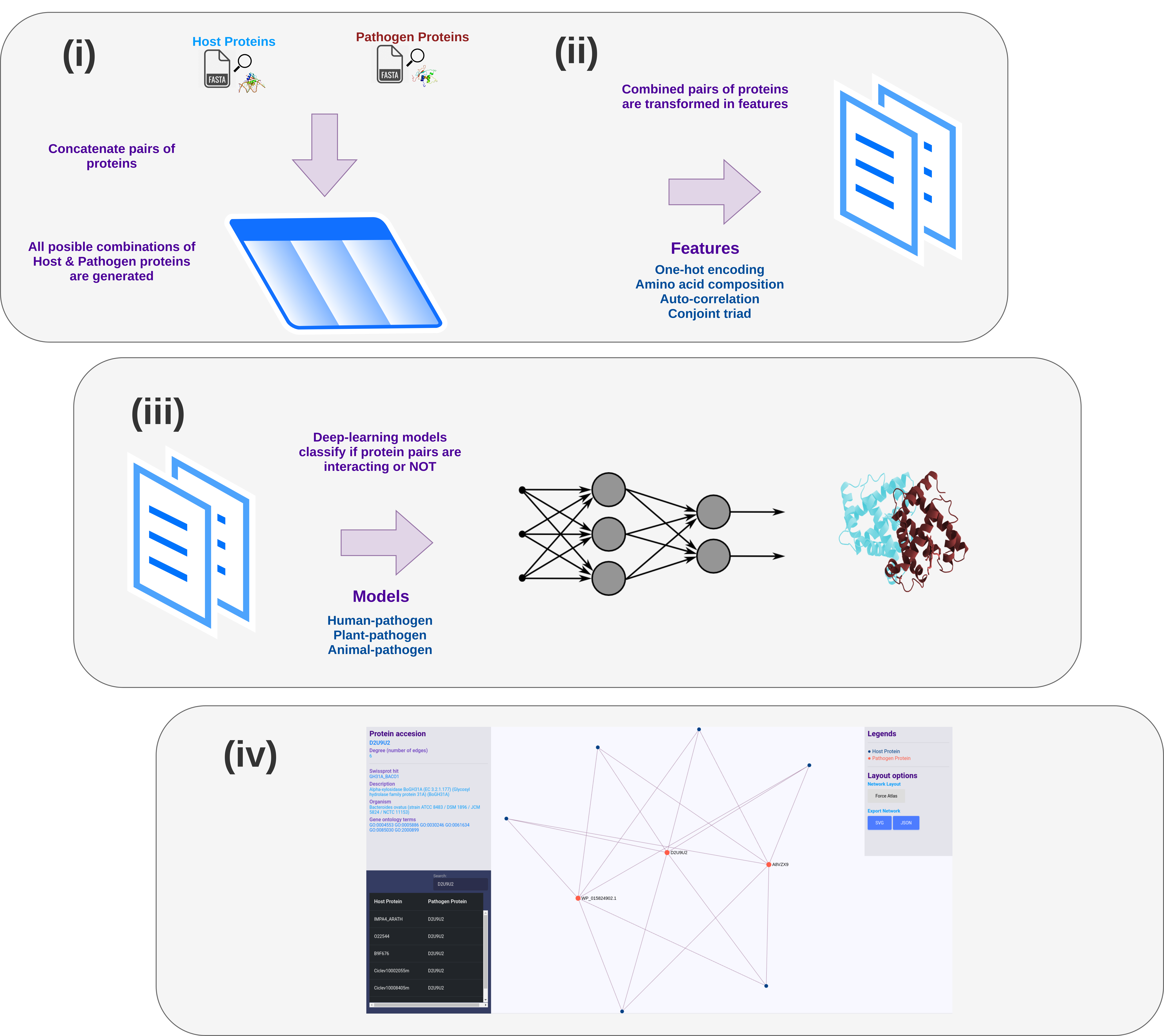